Linear Discriminant Analysis: Meaning of Negative Eigenvalues?
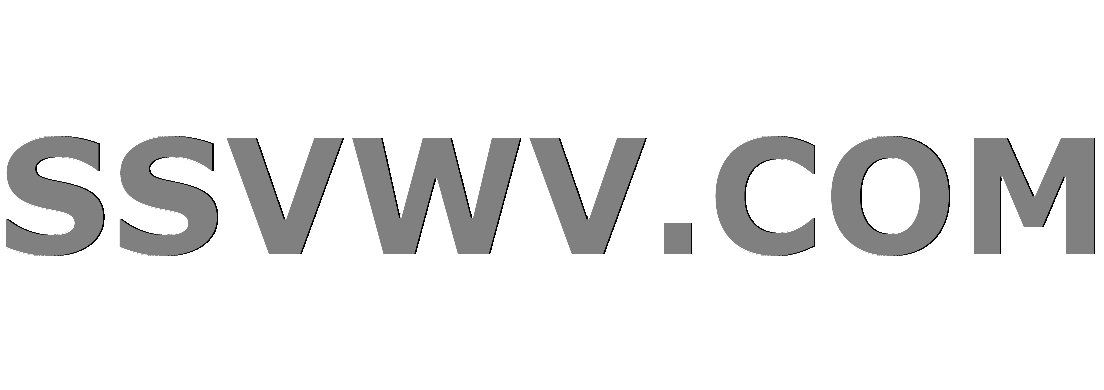
Multi tool use
$begingroup$
In Linear Discriminant Analysis (LDA) we compute two matrices from the data: the between scatter matrix $boldsymbol{S}_b$ and within scatter matrix $boldsymbol{S}_w$. A direction $boldsymbol{w}$ is then considered more discriminative if $(boldsymbol{w}^T boldsymbol{S}_b boldsymbol{w})/(boldsymbol{w}^T boldsymbol{S}_w boldsymbol{w})$ is larger. Therefore, the most $k$ discriminative directions correspond to the top $k$ eigenvectors of the matrix $boldsymbol{S}_b boldsymbol{S}_w^{-1}$.
While the two scatter matrices $boldsymbol{S}_b$ and $boldsymbol{S}_w$ are positive semi-definite, the matrix $boldsymbol{S}_b boldsymbol{S}_w^{-1}$ does not have to be. So I wonder what is the meaning/intuition of negative eigenvalues in this case?
This question arose when I wanted to choose the number $k$ based on what percentage of discriminative information is preserved by the top $k$ eigenvectors. For example, in case of PCA, I could say I want to pick the top $k$ eigenvectors that maintain $90%$ of the variance, i.e. $(sum_{j=1}^k lambda_j)/(sum_{j=1}^n lambda_j) approx 0.9$ ($n$ being the total number of eigenvalues). However, if negative eigenvalues can exist in LDA, how should I modify the PCA idea to achieve similar goal? Should I use $(sum_{j=1}^k lambda_j)/(sum_{j=1}^n lambda_j) approx 0.9$, or $(sum_{j=1}^k lambda_j)/(sum_{j=1}^{n^+} lambda_j) approx 0.9$ ($n^+$ meaning sum over nonnegative eigenvalues only), or $(sum_{j=1}^k lambda_j)/(sum_{j=1}^{n} |lambda_j|) approx 0.9$, or none of these?
Thanks
Golabi
statistics machine-learning
$endgroup$
add a comment |
$begingroup$
In Linear Discriminant Analysis (LDA) we compute two matrices from the data: the between scatter matrix $boldsymbol{S}_b$ and within scatter matrix $boldsymbol{S}_w$. A direction $boldsymbol{w}$ is then considered more discriminative if $(boldsymbol{w}^T boldsymbol{S}_b boldsymbol{w})/(boldsymbol{w}^T boldsymbol{S}_w boldsymbol{w})$ is larger. Therefore, the most $k$ discriminative directions correspond to the top $k$ eigenvectors of the matrix $boldsymbol{S}_b boldsymbol{S}_w^{-1}$.
While the two scatter matrices $boldsymbol{S}_b$ and $boldsymbol{S}_w$ are positive semi-definite, the matrix $boldsymbol{S}_b boldsymbol{S}_w^{-1}$ does not have to be. So I wonder what is the meaning/intuition of negative eigenvalues in this case?
This question arose when I wanted to choose the number $k$ based on what percentage of discriminative information is preserved by the top $k$ eigenvectors. For example, in case of PCA, I could say I want to pick the top $k$ eigenvectors that maintain $90%$ of the variance, i.e. $(sum_{j=1}^k lambda_j)/(sum_{j=1}^n lambda_j) approx 0.9$ ($n$ being the total number of eigenvalues). However, if negative eigenvalues can exist in LDA, how should I modify the PCA idea to achieve similar goal? Should I use $(sum_{j=1}^k lambda_j)/(sum_{j=1}^n lambda_j) approx 0.9$, or $(sum_{j=1}^k lambda_j)/(sum_{j=1}^{n^+} lambda_j) approx 0.9$ ($n^+$ meaning sum over nonnegative eigenvalues only), or $(sum_{j=1}^k lambda_j)/(sum_{j=1}^{n} |lambda_j|) approx 0.9$, or none of these?
Thanks
Golabi
statistics machine-learning
$endgroup$
add a comment |
$begingroup$
In Linear Discriminant Analysis (LDA) we compute two matrices from the data: the between scatter matrix $boldsymbol{S}_b$ and within scatter matrix $boldsymbol{S}_w$. A direction $boldsymbol{w}$ is then considered more discriminative if $(boldsymbol{w}^T boldsymbol{S}_b boldsymbol{w})/(boldsymbol{w}^T boldsymbol{S}_w boldsymbol{w})$ is larger. Therefore, the most $k$ discriminative directions correspond to the top $k$ eigenvectors of the matrix $boldsymbol{S}_b boldsymbol{S}_w^{-1}$.
While the two scatter matrices $boldsymbol{S}_b$ and $boldsymbol{S}_w$ are positive semi-definite, the matrix $boldsymbol{S}_b boldsymbol{S}_w^{-1}$ does not have to be. So I wonder what is the meaning/intuition of negative eigenvalues in this case?
This question arose when I wanted to choose the number $k$ based on what percentage of discriminative information is preserved by the top $k$ eigenvectors. For example, in case of PCA, I could say I want to pick the top $k$ eigenvectors that maintain $90%$ of the variance, i.e. $(sum_{j=1}^k lambda_j)/(sum_{j=1}^n lambda_j) approx 0.9$ ($n$ being the total number of eigenvalues). However, if negative eigenvalues can exist in LDA, how should I modify the PCA idea to achieve similar goal? Should I use $(sum_{j=1}^k lambda_j)/(sum_{j=1}^n lambda_j) approx 0.9$, or $(sum_{j=1}^k lambda_j)/(sum_{j=1}^{n^+} lambda_j) approx 0.9$ ($n^+$ meaning sum over nonnegative eigenvalues only), or $(sum_{j=1}^k lambda_j)/(sum_{j=1}^{n} |lambda_j|) approx 0.9$, or none of these?
Thanks
Golabi
statistics machine-learning
$endgroup$
In Linear Discriminant Analysis (LDA) we compute two matrices from the data: the between scatter matrix $boldsymbol{S}_b$ and within scatter matrix $boldsymbol{S}_w$. A direction $boldsymbol{w}$ is then considered more discriminative if $(boldsymbol{w}^T boldsymbol{S}_b boldsymbol{w})/(boldsymbol{w}^T boldsymbol{S}_w boldsymbol{w})$ is larger. Therefore, the most $k$ discriminative directions correspond to the top $k$ eigenvectors of the matrix $boldsymbol{S}_b boldsymbol{S}_w^{-1}$.
While the two scatter matrices $boldsymbol{S}_b$ and $boldsymbol{S}_w$ are positive semi-definite, the matrix $boldsymbol{S}_b boldsymbol{S}_w^{-1}$ does not have to be. So I wonder what is the meaning/intuition of negative eigenvalues in this case?
This question arose when I wanted to choose the number $k$ based on what percentage of discriminative information is preserved by the top $k$ eigenvectors. For example, in case of PCA, I could say I want to pick the top $k$ eigenvectors that maintain $90%$ of the variance, i.e. $(sum_{j=1}^k lambda_j)/(sum_{j=1}^n lambda_j) approx 0.9$ ($n$ being the total number of eigenvalues). However, if negative eigenvalues can exist in LDA, how should I modify the PCA idea to achieve similar goal? Should I use $(sum_{j=1}^k lambda_j)/(sum_{j=1}^n lambda_j) approx 0.9$, or $(sum_{j=1}^k lambda_j)/(sum_{j=1}^{n^+} lambda_j) approx 0.9$ ($n^+$ meaning sum over nonnegative eigenvalues only), or $(sum_{j=1}^k lambda_j)/(sum_{j=1}^{n} |lambda_j|) approx 0.9$, or none of these?
Thanks
Golabi
statistics machine-learning
statistics machine-learning
edited Dec 14 '18 at 22:33
Golabi
asked Dec 14 '18 at 21:51
GolabiGolabi
727
727
add a comment |
add a comment |
0
active
oldest
votes
Your Answer
StackExchange.ifUsing("editor", function () {
return StackExchange.using("mathjaxEditing", function () {
StackExchange.MarkdownEditor.creationCallbacks.add(function (editor, postfix) {
StackExchange.mathjaxEditing.prepareWmdForMathJax(editor, postfix, [["$", "$"], ["\\(","\\)"]]);
});
});
}, "mathjax-editing");
StackExchange.ready(function() {
var channelOptions = {
tags: "".split(" "),
id: "69"
};
initTagRenderer("".split(" "), "".split(" "), channelOptions);
StackExchange.using("externalEditor", function() {
// Have to fire editor after snippets, if snippets enabled
if (StackExchange.settings.snippets.snippetsEnabled) {
StackExchange.using("snippets", function() {
createEditor();
});
}
else {
createEditor();
}
});
function createEditor() {
StackExchange.prepareEditor({
heartbeatType: 'answer',
autoActivateHeartbeat: false,
convertImagesToLinks: true,
noModals: true,
showLowRepImageUploadWarning: true,
reputationToPostImages: 10,
bindNavPrevention: true,
postfix: "",
imageUploader: {
brandingHtml: "Powered by u003ca class="icon-imgur-white" href="https://imgur.com/"u003eu003c/au003e",
contentPolicyHtml: "User contributions licensed under u003ca href="https://creativecommons.org/licenses/by-sa/3.0/"u003ecc by-sa 3.0 with attribution requiredu003c/au003e u003ca href="https://stackoverflow.com/legal/content-policy"u003e(content policy)u003c/au003e",
allowUrls: true
},
noCode: true, onDemand: true,
discardSelector: ".discard-answer"
,immediatelyShowMarkdownHelp:true
});
}
});
Sign up or log in
StackExchange.ready(function () {
StackExchange.helpers.onClickDraftSave('#login-link');
});
Sign up using Google
Sign up using Facebook
Sign up using Email and Password
Post as a guest
Required, but never shown
StackExchange.ready(
function () {
StackExchange.openid.initPostLogin('.new-post-login', 'https%3a%2f%2fmath.stackexchange.com%2fquestions%2f3039922%2flinear-discriminant-analysis-meaning-of-negative-eigenvalues%23new-answer', 'question_page');
}
);
Post as a guest
Required, but never shown
0
active
oldest
votes
0
active
oldest
votes
active
oldest
votes
active
oldest
votes
Thanks for contributing an answer to Mathematics Stack Exchange!
- Please be sure to answer the question. Provide details and share your research!
But avoid …
- Asking for help, clarification, or responding to other answers.
- Making statements based on opinion; back them up with references or personal experience.
Use MathJax to format equations. MathJax reference.
To learn more, see our tips on writing great answers.
Sign up or log in
StackExchange.ready(function () {
StackExchange.helpers.onClickDraftSave('#login-link');
});
Sign up using Google
Sign up using Facebook
Sign up using Email and Password
Post as a guest
Required, but never shown
StackExchange.ready(
function () {
StackExchange.openid.initPostLogin('.new-post-login', 'https%3a%2f%2fmath.stackexchange.com%2fquestions%2f3039922%2flinear-discriminant-analysis-meaning-of-negative-eigenvalues%23new-answer', 'question_page');
}
);
Post as a guest
Required, but never shown
Sign up or log in
StackExchange.ready(function () {
StackExchange.helpers.onClickDraftSave('#login-link');
});
Sign up using Google
Sign up using Facebook
Sign up using Email and Password
Post as a guest
Required, but never shown
Sign up or log in
StackExchange.ready(function () {
StackExchange.helpers.onClickDraftSave('#login-link');
});
Sign up using Google
Sign up using Facebook
Sign up using Email and Password
Post as a guest
Required, but never shown
Sign up or log in
StackExchange.ready(function () {
StackExchange.helpers.onClickDraftSave('#login-link');
});
Sign up using Google
Sign up using Facebook
Sign up using Email and Password
Sign up using Google
Sign up using Facebook
Sign up using Email and Password
Post as a guest
Required, but never shown
Required, but never shown
Required, but never shown
Required, but never shown
Required, but never shown
Required, but never shown
Required, but never shown
Required, but never shown
Required, but never shown
TyopZtBbXRvCAE7ok24GXmdIqzAoWAf